As mentioned in the introduction to behavioral economics, biased beliefs are beliefs affected by context at the formation of the belief. The example presented in the introduction is simple: a person who is hungry today expects to have a similar hunger level a day or more in the future (which is likely untrue). At that moment, they choose a filling snack according to their current hunger level rather than their probable future hunger level (Read & van Leeuwen, 1998).
Importantly, biased beliefs are consistent and predictable differences between actions and consequences. Models have been created to understand these systematic differences and attempt to predict the actions of agents with biased beliefs. There are many examples of biased beliefs and how they can affect decision-making, some of which will be discussed in the following sections.
Biased Beliefs Examples
1. Projection Bias
Projection bias is perhaps one of the most widely known and discussed of the examples of biased beliefs. In a seminal paper published in The Quarterly Journal of Economics, Loewenstein, O’Donoghue, and Rabin establish a model for projection bias, discuss evidence of projection bias, and explore its implications in predicting future utility (Loewenstein et al., 2003). In their words, projection bias is that “people tend to understand qualitatively the directions in which their tastes will change, but systematically underestimate the magnitudes of these changes”.
In fact, the example given in the introduction is a clear example of projection bias. Other simple examples that Loewenstein et al. present are: the tendency to choose unreasonably warm vacation destinations when planning the vacation in the winter, the tendency to order more food at the beginning of a meal than one will likely eat, and an underestimation of the power of addiction by people not addicted to cigarettes. Additional examples include the tendency of people to buy more convertibles on warmer days, to purchase homes with pools on hotter summer days, to purchase more food at the grocery store when hungry than when full, and to purchase more winter coats on exceptionally cold winter days (Busse et al., 2012; Mela et al., 1996; Conlin et al., 2007).
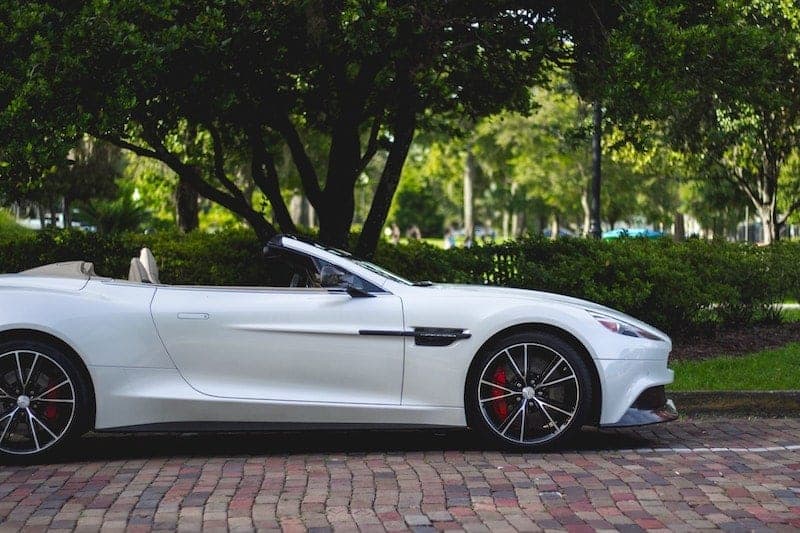
While there are many variations of the projection bias due to weather and hunger, another interesting example is the effect of projection bias on medical decision making (Loewenstein, 2005). Loewenstein discusses several topics, including life support for patients with depression, adherence, medication for pain, end-of-life care, and even physician training. In the adherence example, Loewenstein states that people will have excessive reactions to pain in the moment but insufficient reactions to those feelings otherwise. “Projection bias, therefore, is likely to pose problems when it comes to people testing for or taking preventative measures to avoid, conditions that don’t cause immediate fear. It is also likely to pose a problem when it comes to adherence to drug regimens for conditions with intermittent symptoms”. A common example of a lack of adherence occurs with antibiotics – doctors often struggle to ensure that patients take the drug for the allotted period of time since many patients will stop taking the antibiotic after their symptoms cease or subside.
2. Hot Hand Fallacy
The hot hand fallacy is a well-known biased belief that has its roots in basketball and probability. Thomas Gilovich first introduced the idea of the hot hand fallacy in 1985: “basketball players and fans alike tend to believe that a player’s chance of hitting a shot are greater following a hit than following a miss on the previous shot” (Gilovich et al., 1985). However, after conducting analyses on free-throw data from the Philadelphia 76ers, Gilovich and his team found no evidence to support the idea of the hot hand fallacy.
In fact, Gilovich’s evidence even seemed to indicate the opposite of a hot-hand fallacy in some cases. For most players, the probability of making a basket was actually lower after having just made a basket than having just missed. Additionally, the probability of making a shot after a streak of making it was lower than after a streak of misses. Gilovich concludes the paper with a comment on why the hot hand fallacy exists: “the belief in the hot hand and the ‘detection’ of streaks in random sequences is attributed to a general misconception of chance according to which even short random sequences are thought to be highly representative of their generating process”. Essentially, the hot hand fallacy is an example of a biased belief based in misunderstanding of probabilistically independent events, and it has applications outside the realm of basketball.
3. Gambler’s Fallacy
The gambler’s fallacy is often discussed and analyzed with the hot hand fallacy. Another biased belief, the gambler’s fallacy can be thought of almost as the opposite of the hot hand fallacy – it “is the belief that the probability of an event is lowered when that event has recently occurred, even though the probability of the event is objectively known to be independent from one trial to the next” (Clotfelter & Cook, 1993).
For example, if a coin is flipped five times in a row and comes up heads every time, the gambler’s fallacy would leave one to believe that tails is “overdue” and that the next flip has a higher probability of coming up tails. However, that reasoning is incorrect because, as long as the coin is a fair coin, it has an equal probability of coming up heads or tails on the next flip, regardless of how it has come up in the past.
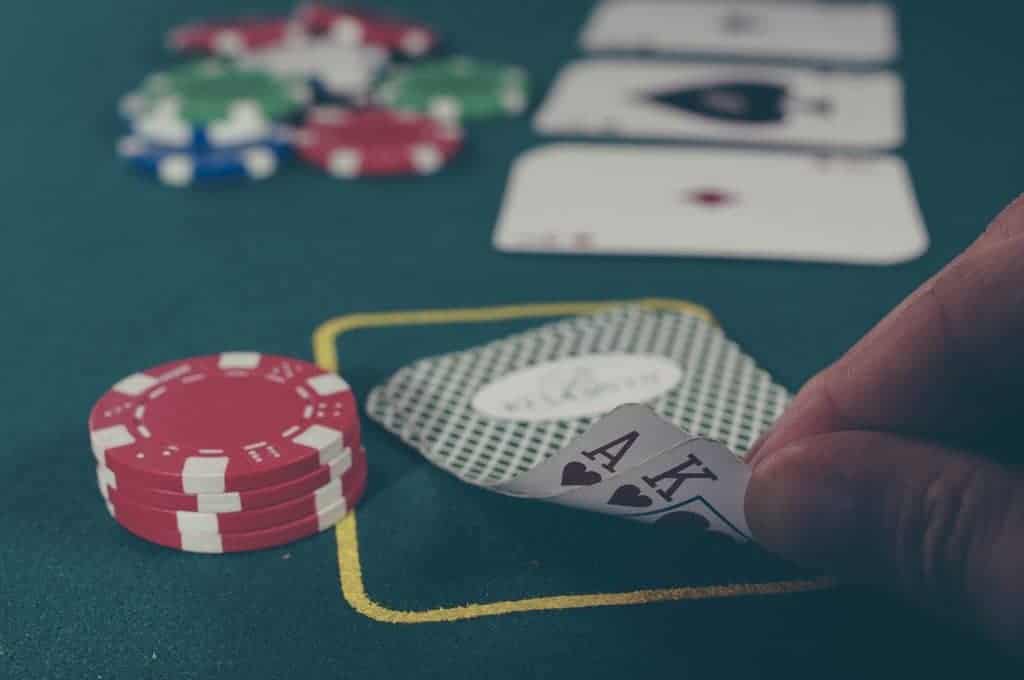
Both the gambler’s fallacy and the hot hand fallacy are examples of representativeness heuristics. These heuristics are essentially rules of thumb that people use to make a judgment about the probability of what’s coming next based on a previous sequence. Additionally, the law of small numbers is an important concept for these two fallacies. Tversky and Kahneman first wrote about the law of small numbers in 1971; they said that people tend to believe a small sample drawn from a random population is highly representative of the overall population. More specifically, people “expect any two samples drawn from a particular population to be more similar to one another and to the population than sampling theory predicts, at least for small samples (Tversky & Kahneman, 1971).